Spam Filtering Using Machine Learning: A Detailed Guide for IT Services and Security Systems
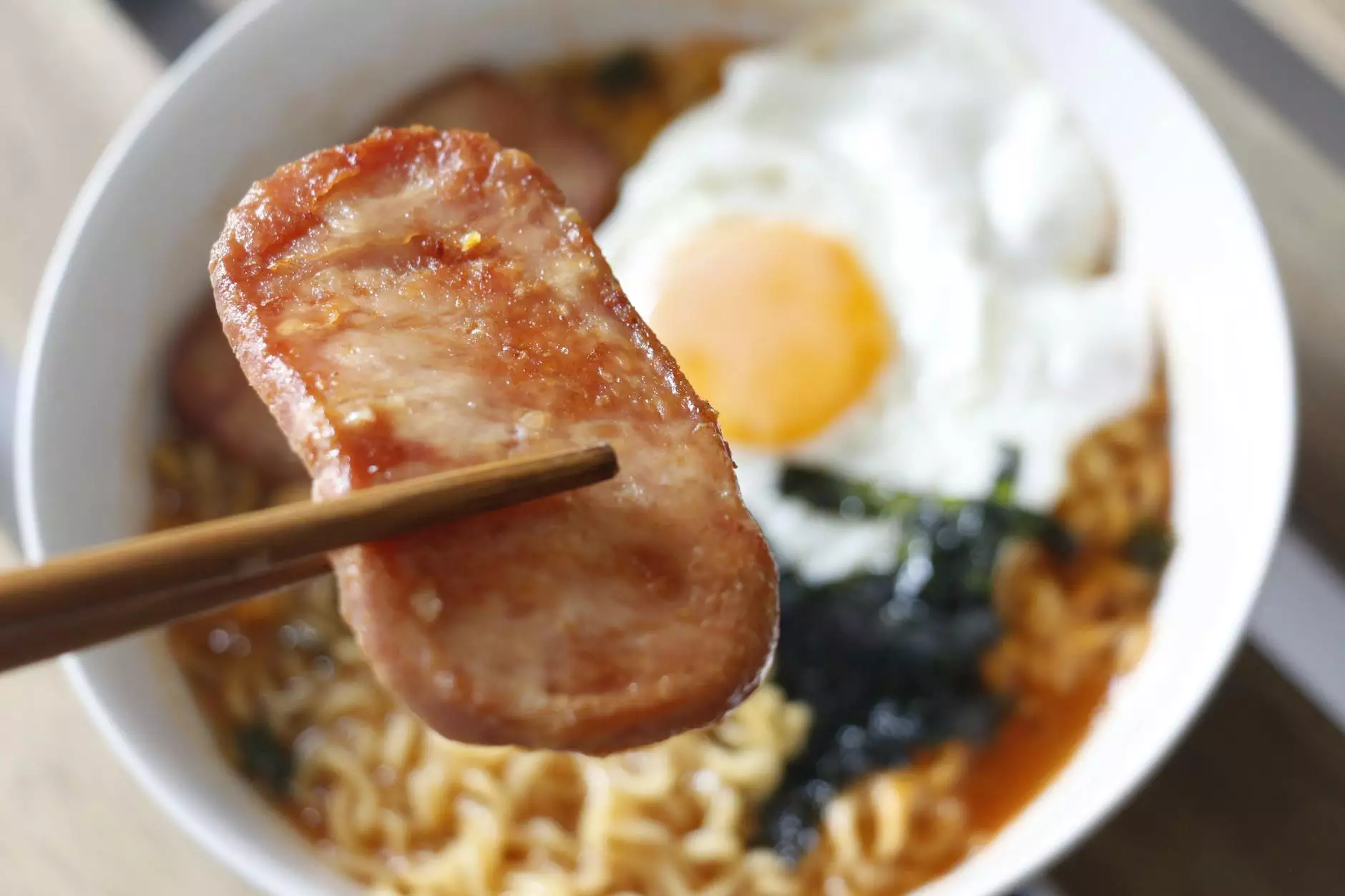
In today's digital age, businesses face an incessant barrage of unwanted emails, often referred to as spam. This can stem from promotional materials to malicious threats such as phishing attacks. As businesses like Spambrella focus on IT services and computer repair, the implementation of advanced techniques like spam filtering using machine learning has become indispensable.
Understanding Spam Filtering
Spam filtering is the process of identifying undesired email content with the intent to block or redirect it from a user's inbox. Machine learning enhances this basic process by utilizing algorithms that can learn from the data patterns and improve over time. Here’s a closer look at how it works:
How Spam Filters Work
Traditionally, spam filters relied on predefined rules and blacklists to pinpoint unwanted emails. However, machine learning algorithms take a different approach, focusing on the content analysis and user behavior patterns. Here’s how they function:
- Training Phase: The algorithm analyzes a set of emails—both spam and legitimate—to learn the distinguishing characteristics.
- Feature Extraction: It identifies unique features such as keywords, sender's addresses, and email metadata that often indicate spam.
- Classification: New incoming emails are classified based on the learned features, determining the likelihood of being spam.
- Continuous Learning: As it processes more emails, the algorithm refines its parameters for greater accuracy.
The Role of Machine Learning in Spam Filtering
Machine learning has revolutionized multiple sectors, including IT services and the implementation of security systems. The advantages of leveraging machine learning for spam filtering include:
1. Enhanced Accuracy
Machine learning filters can continuously improve their understanding of what constitutes spam. By constantly updating their understanding based on user feedback and evolving attacks, they significantly reduce false positives and negatives. This results in a cleaner inbox and enhanced user experience.
2. Adaptive Learning
Unlike static filters, machine learning models adapt to new types of spam as they emerge. With spam tactics changing frequently, an effective filter must evolve correspondingly. These systems learn from every interaction, making them more effective over time.
3. Lower Operational Costs
With a reliable machine learning spam filter in place, businesses like Spambrella can save on costs associated with manual email monitoring and user complaints. Automating the spam filtering process allows IT personnel to focus on more strategic initiatives.
Key Techniques in Machine Learning Spam Filtering
To implement an effective spam filtering system using machine learning, several techniques are commonly used:
1. Supervised Learning
Supervised learning involves training an algorithm on a labeled dataset, where the algorithm learns to differentiate spam from legitimate emails based on features learned during training.
2. Unsupervised Learning
In scenarios where labeled data is limited, unsupervised learning can help identify patterns and cluster similar emails together, which can be indicative of spam.
3. Natural Language Processing (NLP)
NLP plays a crucial role in understanding and interpreting the content of emails. By evaluating the language and context, NLP can help identify potential spam messages effectively.
The Importance of Regular Updates
The landscape of spam is continuously evolving, and so must your spam filtering system. Regular updates that incorporate the latest data and adjust for novel spam techniques are vital:
1. Keeping Up with Spam Trends
By analyzing the latest spam trends, filtering algorithms can adjust their models to include new identifiers for spam messages, making it harder for spammers to bypass defenses.
2. User Feedback Systems
Incorporating user feedback can enrich the dataset that algorithms learn from. By understanding user marking of spam, the system can refine its filters based on actual user interactions, leading to better accuracy.
Implementing Machine Learning Spam Filters
For businesses looking to integrate spam filtering using machine learning into their existing systems, there are several approaches to consider:
1. Cloud-Based Solutions
Many organizations opt for cloud-based spam filtering solutions which allow for easy integration, scalability, and access to powerful machine learning resources.
2. On-Premise Solutions
For businesses with strict data privacy concerns, on-premise solutions offer more control over data while utilizing local resources for spam filtering processes.
3. Hybrid Models
A hybrid approach can provide the best of both worlds by combining cloud-based and on-premise solutions, optimizing for performance and control.
Future of Spam Filtering Using Machine Learning
The future of spam filtering using machine learning is likely to see even more sophisticated techniques that incorporate cutting-edge advancements:
1. Integration with AI Systems
The convergence of machine learning with broader AI systems will enhance spam filtering capabilities, allowing for more nuanced decision-making and threat detection.
2. Regulatory Compliance
As data protection regulations become stricter, spam filtering systems will need to incorporate features that ensure compliance with laws such as GDPR, making privacy a stronger focal point.
3. Personalized Filtering
Future models may leverage even more personalized filtering capabilities, where user preferences and interactions inform the spam detection process uniquely for each user.
Conclusion
In conclusion, spam filtering using machine learning is changing the way businesses handle unwanted email communications. With enhanced accuracy, adaptive learning, and cost savings, it’s clear that organizations like Spambrella can greatly benefit from implementing these advanced techniques in their IT services and security systems.
As the digital landscape continues to evolve, staying ahead of spam threats through cutting-edge technologies will be essential for maintaining operational efficiency and safeguarding sensitive data.
Call to Action
Are you ready to take your email security to the next level? Contact Spambrella today to learn more about integrating state-of-the-art spam filtering using machine learning into your business operations.